How human translators are coping with competition from powerful AI
Productivity is up and real wages are down, but humans are still in the game.
You can listen to an audio version by clicking the button above or by subscribing to Understanding AI with your favorite podcast app. I used AI software (specifically Descript’s Overdub) to synthesize my voice and included actual audio of sources I quoted in the piece. This took a couple hours of work, so if you would like me to continue doing this with future articles, please let me know by leaving a comment or responding by email. Thanks!
When ChatGPT was released last fall, a lot of white-collar workers had a chilling thought: “could this technology do my job?” A May survey by CNBC and SurveyMonkey found that almost a quarter of workers fear losing their jobs to AI in the next few years, including 51 percent of workers in advertising and marketing and 46 percent of those in business support and logistics.
But for one white-collar profession—translators—this stopped being a theoretical question years ago. ChatGPT is based on a neural network architecture called the transformer that was first used by Google for machine translation in 2017. So competition from AI has been an everyday reality for human translators for more than five years.
Marc Eybert-Guillon started his career as a translator in 2017. In 2020, he founded From the Void, a firm that helps video game makers localize their games for foreign markets.
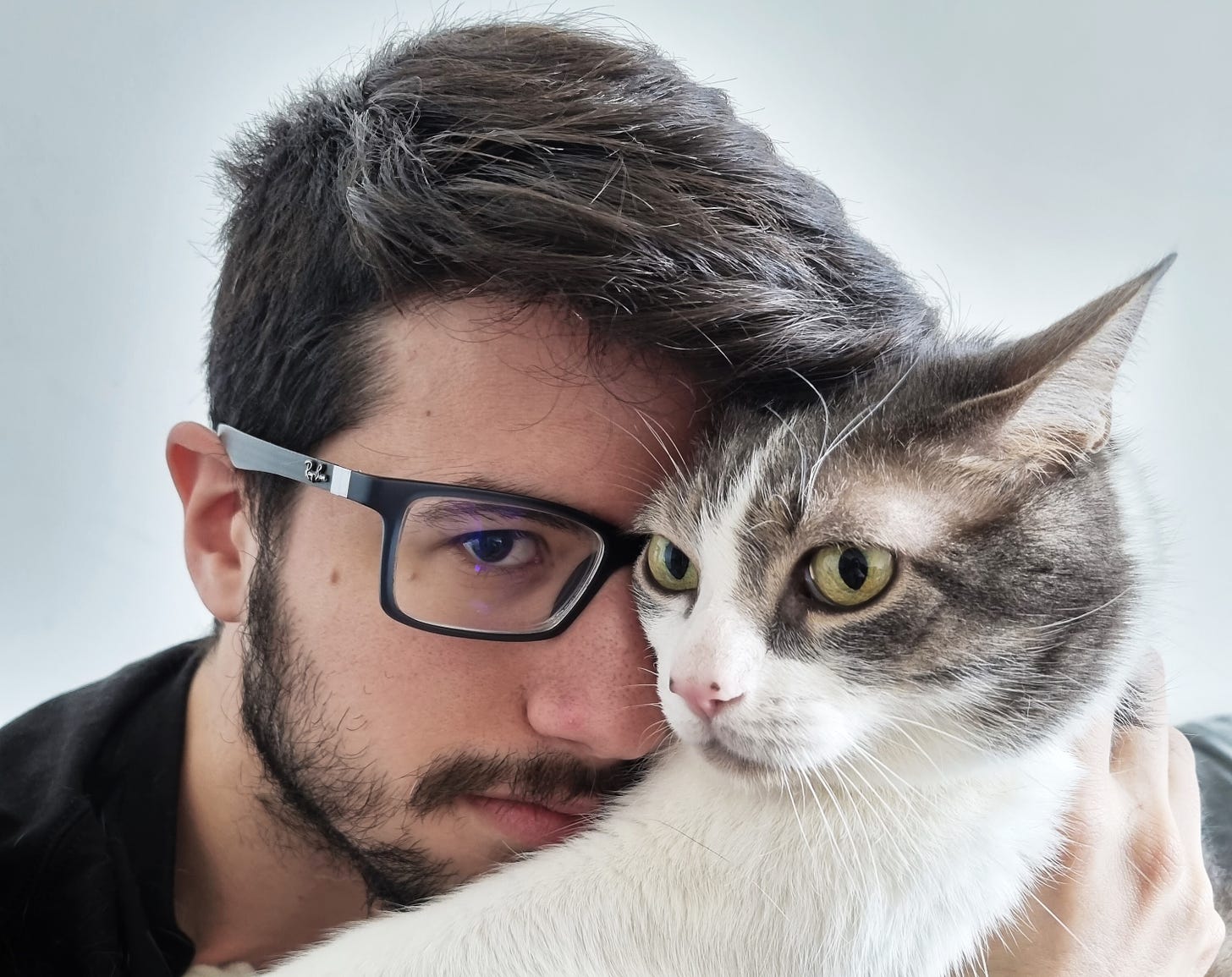
“It's that meme of the guy with the noose around his neck,” Eybert-Guillon told me. The condemned man looks over at the guy standing next to him on the gallows and asks “first time?”
“We’ve been ‘in danger’ of being taken over by AI for 10 years now and it still hasn’t happened,” Eybert-Guillon said. “But we keep getting told that it’s going to happen.”
There are two big reasons AI hasn’t put many human translators out of work. First, human translators still do a better job in specialized fields like law and medicine. Translation errors in these fields can be very expensive, so clients are willing to pay extra for a human-quality translation.
Second, there has been rapid growth in hybrid translation services where a computer produces a first draft and a human translator checks it for errors. These hybrid services tend to be about 40 percent cheaper than a conventional human translation, and customers have taken advantage of that discount to translate more documents. Translators get paid less per word, but they’re able to translate more words per hour.
But while AI software has not put human translators out of work the way pessimists might have predicted, this isn’t an entirely positive story for translators either.
“I think rates for translators have stayed largely the same for 10 or 12 years,” said Mark Hemming, a translator in the United Kingdom. “I think it is harder to get work now. I think it's harder to get well paid work as well.”
The main lesson, though, is that workers in other industries don’t need to worry about AI taking over their jobs overnight. Automation is a gradual process that plays out over decades, not years or months. And there are plenty of ways human workers can adapt.
Some translators do work AI can’t
Barbara Leon is a freelance translator in Spain. She focuses on translating legal documents like contracts, trusts, immigration records, and divorce agreements between English and Spanish. There’s a robust market for human translation of documents like this because errors can be expensive. Moreover, some of the documents Leon produces are submitted to courts or government agencies that require sign-off by an expert human translator.
To translate a legal document accurately, Leon doesn’t just need to be an expert on the English and Spanish languages. She also needs a deep understanding of legal systems on both sides of the Atlantic.
“If you put ‘trust’ in ChatGPT it's going to translate it to confianza,” Leon said. “But that's not what it means.” In reality, Leon says, there are 20 or 30 different ways to translate the legal concept of a trust to Spanish. Figuring out which meaning to use in any given sentence requires a sophisticated understanding of American law, Spanish law, and the context of the specific document she is translating.
It’s a similar story for Marc Eybert-Guillon’s work localizing video games. His firm provides translation for a wide variety of text, from character dialog to the labels on in-game items like weapons or magic potions. Often he needs to translate a single word or short phrase—too little context for an unambiguous translation.
To gain the necessary insight, he may play a pre-release version of the game or ask the game’s developers to explain it. This kind of exploration is beyond the capabilities of software like Google Translate or ChatGPT.
There’s also an important element of creativity to video game design, Eybert-Guillon argues. Machine translation software might provide a literal translation of a word like “dagger” or “pistol” when a more whimsical or obscure term might be a better fit. It’s hard to provide a good translation without understanding the gaming culture in a particular target market.
Mark Hemming told me about a friend who specializes in doing translations related to outdoor sports like climbing, mountain biking, and skiing. A company selling forks for mountain bikes might hire her to translate its product descriptions and installation instructions. Translators who aren’t into outdoor sports may not know the right vocabulary—and ChatGPT probably wouldn’t either.
In short, when you look closely at the market for translators, what you find is actually a bunch of distinct sub-markets. The skills required to translate text in a video game are different from those needed to translate a legal document or the installation instructions for mountain bike parts. Each of these translation tasks requires context beyond the four corners of the document being translated—context that a computer program likely doesn’t have. So clients that care about quality are willing to pay extra for an experienced human to do the job.
Lower cost drives higher demand
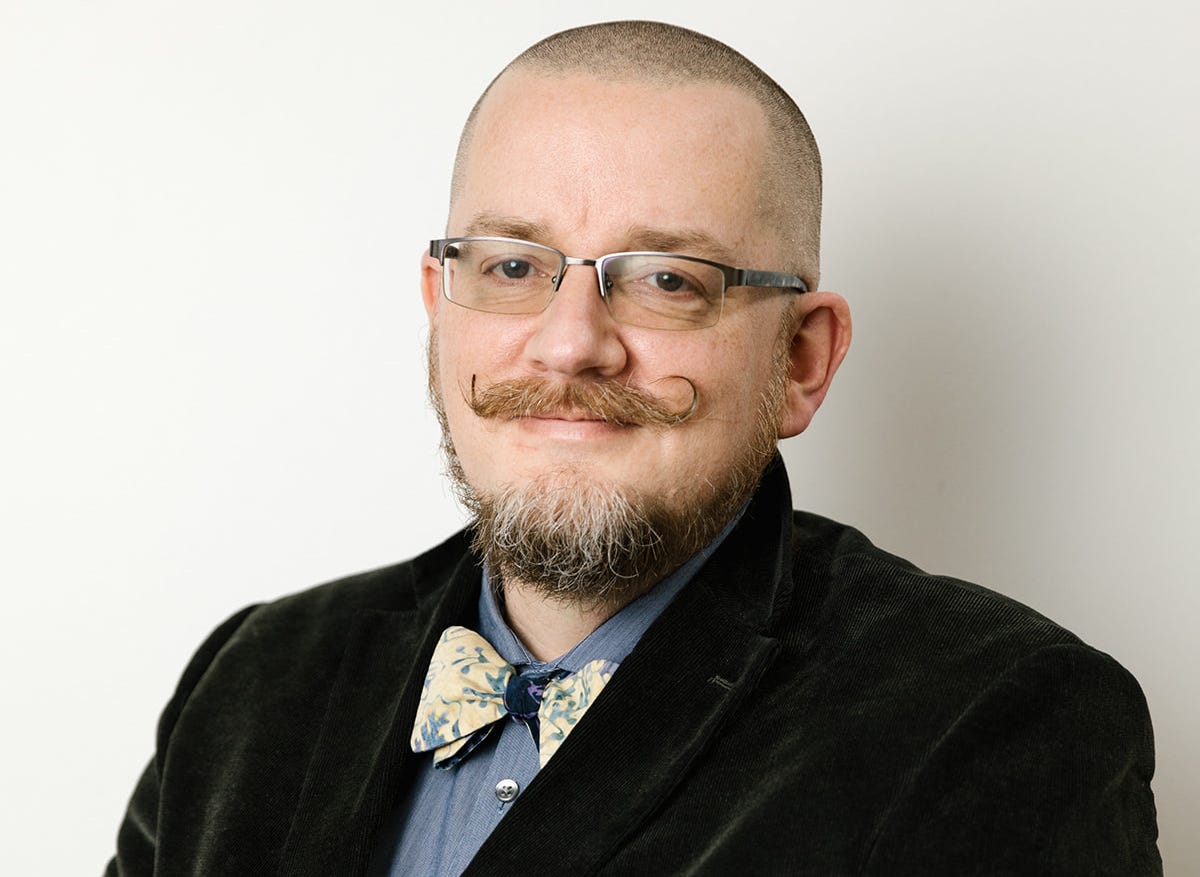
While some customers are willing to pay a premium for high quality, there are plenty of other customers who are perfectly willing to accept lower quality work if it saves them money.
“A lot of use cases don't demand perfect fluency or accuracy,” said Arle Lommel, an analyst at the market research firm CSA, in a recent interview. “Obviously probably people would prefer something done by the best translator in the world, but usually the alternative to that is no translation, and that doesn't benefit anyone.”
So while there continues to be robust demand for fully human translation, there’s also growing demand for a hybrid approach where a computer generates the first draft and a person checks it for accuracy.
“The process of editing the output of machine translation was initially perceived to be viable only for very specific types of content,” said Alon Lavie, a professor at Carnegie Mellon and an executive at the machine translation company Unbabel. “And now I think the tide has kind of turned and most types of content, most use cases, I think it's clear that just editing the output of automated translation is more cost-effective, faster, and more efficient to do.”
Lommel estimates that this kind of service typically costs about 60 percent as much as a traditional human translation. And many clients take advantage of this discount to have more text translated.
While translators don’t like that discount, Lommel argues that the technology will enable them to translate more words per hour. In the long run, this might even lead to higher incomes.
Many of the translators I spoke to remain skeptical of this approach. They told me that machine translations are often so bad that it takes more work to fix them than it would have taken to produce a translation from scratch. Some found the experience so frustrating that they’ve stopped accepting this kind of work.
Yet Lommel says his firm’s data shows that machine-augmented translation is growing faster than conventional human translation. Because customers who buy cheaper semi-automated translation services don’t care as much about quality, these services can probably get away with using less skilled translators.
I see a parallel here to the way smartphones have changed the taxi industry over the last 15 years. Before the advent of smartphones, driving a taxi required extensive knowledge of local streets. Indeed, New York once required cabbies to pass a geography test before they could get a license.
Smartphones changed that. The Uber and Lyft apps supplied turn-by-turn directions that allowed unskilled drivers like me to drive without any special training or experience. That was not a positive development for existing taxi drivers because it devalued their hard-won navigational skills. But it was good for consumers, who got more ubiquitous and affordable taxi services. And it was also good for many new drivers—especially those who used Uber or Lyft as a temporary or part-time source of income.
If this analogy is right, then I would expect translation software to lower barriers to becoming a translator, which may reduce both the quality of translations and the average wages of translators. I can understand why this might drive experienced translators crazy. But it’s also easy to see why many customers would see it as a good trade. As Lommel says, a low-quality translation is often better than no translation at all.
Data from the Bureau of Labor Statistics is consistent with this interpretation. The number of translators and interpreters rose 19 percent between 2010 and 2021: from 58,400 to 69,400. But their median income, adjusted for inflation, fell by 8 percent. In 2010, a typical translator made $60,357 (in 2023 dollars). By 2021 it was $55,483.
CSA estimates that global revenue from translation, interpreting, and other language services grew by 23 percent (in inflation-adjusted dollars) between 2012 and 2022. Yet when CSA surveyed translators on the state of the industry, many of them were quite negative about their career prospects.
“It's a declining business,” wrote one translator in response to a CSA survey. “I still make decent money because I am a specialist (finance, tech, agriculture). I am setting up a new, completely unrelated business and hope to stop translating as this business replaces my current income.”
Automation is good for consumers
The advent of machine translation has not been catastrophic for human translators, but machine translation software does seem to be putting downward pressure on wages. And it’s easy to imagine that pressure intensifying in the coming years as AI technology improves further.
At the same time, machine translation has been unambiguously positive for consumers of translated content. Multinational companies, government agencies, and other organizations collectively produce billions of documents every year and only a tiny fraction of these are ever translated into even one other language. There are dozens of languages around the world with millions of native speakers.
This means that there is a ton of value that could be created by translating more documents into more languages. For example, companies tend to see a big increase in sales in a country when they translate their marketing materials into that country's native language. So cheaper translation services would enable a lot of companies to expand the market for their products around the world.
So when thinking about the impact of automation on workers, it’s important to remember that every worker is also a consumer. We all benefit when automation in other industries lowers the cost of the goods and services we buy. And while having your own industry automated isn’t fun, it’s usually not catastrophic.
Pessimists will counter that this time is different—that AI will soon become so sophisticated that it will put all human translators out of a job. Arle Lommel doesn’t buy it.
“My expectation is that so much stuff needs to be translated, that there's always going to be a role for the human, especially when you’re introducing new technologies and new topics,” Lommel said. “Machines are always stuck at some point in the past in terms of what they can do. Language is changing, and you need humans because the machines can only go off what’s been done before, not what is new.”
Maybe you find that convincing or maybe you don’t. But either way the pace of change matters.
If the introduction of neural translation software in 2017 had put every translator out of a job in 2018, that would have been a huge hardship for them and their families. On the other hand, if steady progress in machine translation technology leads to a gradual decline in translator employment over the next 10 to 20 years, that’s a more manageable situation. That will give older translators time to reach retirement age and younger translators time to switch careers.
If you’re worried that your own profession will get automated by AI software in the coming years, I hope that the story of translators helps to reduce your anxiety level. It seems very possible that AI software will start to automate other white-collar jobs over the next five to 10 years. But the key word here is start.
The first iterations of the software likely won’t be very good, leaving plenty of tasks in human hands. Early AI systems in your profession may increase demand for human workers to oversee and correct the work of automated systems. Even if that doesn’t happen, job losses are likely to play out over the course of decades rather than years, giving alert workers plenty of time to adapt.
I have written about this myself from the perspective of a human translator: https://alakasa.substack.com/p/how-will-gpt-4-affect-white-collar
I think the most crucial thing not noted here is that there are still many non-AI translation jobs available simply for the reason that not all companies want their confidential and proprietory data to become some AI/machine translation company's property and grist for their algorithms via being fed to a MT application, such as by some unaware and careless translator.
This article misses a few crucial points.
Firstly, with the advent of generative AI like ChatGPT, we are likely to see more and more non-native speakers confidently producing their own texts in foreign languages or post-editing MT output themselves. The demand for translations may well decrease considerably – especially for translations into and out of English.
Secondly, the efficiency of MT is a myth.
While some translators find that editing MT output increases their productivity, many experienced translators find that post-editing takes them more time than a normal translation (see e.g. Kränzler, Artificial Intelligence in Technical Translation, table 2 on page 5, 201217_sof8_ai-in-technical_translation.pdf). Post-editing MT output requires comparing two existing texts, assessing the quality of the target text and, if found to be necessary, editing it. This activity is more complex than grasping the meaning of just one text and rendering it in the target language in the grammatically and stylistically most appropriate way. Ironically, as MT technology gets better, it produces more plausible output and spotting mistakes in the MT output requires more concentration rather than less.
Any claims about the efficiency of MT in comparison to professional human translation appear even more doubtful if you consider the enormous amount of resources that go into building and operating MT systems – not just in terms of computing power (hardware and electricity) and data, but also in terms of brain power for programming and training.
So far, it is not clear how to make money with artificial intelligence based on Large Language Models. Still, investors betting on what they hope will prove to be a revolutionary technology are pouring huge amounts of money into this technology – disrupting and distorting the translation market.
MT systems are expensive. Training and fine-tuning them is costly. Language Services Providers (LSPs) feel the need to invest in MT to stay competitive, but struggle to recoup their costs – last, not least because their customers have come to expect paying 40% less for translations. The only cost factor left for making savings appears to be translators’ pay. The beauty of being hit with a 30-40% rate reduction is that 10% inflation barely register.
By introducing new benchmarks (“adequate” or “fit for purpose” translations) LSPs’ (ab)use of MT has driven down translation quality and prices as well as translators’ rates and job satisfaction. Translators are considering career changes and students’ interest in translation courses is in decline.
This development could perhaps be halted if translators’ rates were not determined depending on how the target text is produced, but depending on the expected quality of the translation. According to the relevant ISO standard 18587:2017 “full post-editing” is the “process to obtain a product comparable to a product obtained by human translation“.
On principle, a product of the quality of a human translation should always be remunerated like a human translation. Whether translating from scratch or doing full post-editing, professional translators deliver what MT will never be able to do. Only humans are able to grasp and render the full meaning of a text, to explain their translation decisions and to accept liability for their mistakes. It should go without saying that their work needs to be remunerated accordingly.
Few people would expect their hairdresser to charge them less if they supplied him with a pair of scissors – or presented him with the results of a robot’s first attempt at cutting their hair. If translation is to remain a sustainable profession, translators need to take a page from the hairdressers’ book.